Distracted Driver Posture and Head Position Identification via m-CNNs.
Distracted Driver Detection GitHub
Paper, results and discussions can be found here
This work is initially inspired by the State Farm’s Distracted Driver Detection Competition and by the work done by Eraqi et al.
The aim of this work is to go beyond the initial limitations of the above approaches by intoroducing second camera to monitor driver’s frontal and facial features along with his/her posture. The approach is based on individual image classifications on ResNet50 models which are then used as an input for final model based on multi-modal approach. More information on the approach, dataset generation, and results can be found here
Sample images of self gathered dataset:
During the Fall 2019 in my junior year, I have taken a Machine Learning with Applications class. Although I already had some prior knowledge of ML and DL algorithms learned in my spare time both from books and MOOCs, I wanted to get more exposure to real-world applications. For my midterm project, I have found a challenging task for Distracted Driver identification. For this purpose, I used the transfer learning technique to use a pre-trained ResNet model on the ImageNet dataset to save time, computation resources, and preserve a generalization property. As was shown further the total accuracy of 91% was successfully achieved on driver activity classification. However, I found distraction classification only from posture images a limitation of the work and proposed to the professor and my team to additionally use driver frontal images for classification. For this purpose, I suggested collecting our own synchronized two camera dataset. Finally, we collected data for 11 people on 7 different classes. Training for separate images was conducted on my modified ResNet architecture separately which were finally concatenated and followed by other fully connected layers. This is usually called a multimodal approach. The accuracies obtained are 92% and 80% for both frames, but the multimodal approach has shown worse accuracy of 84%.
Some results (full results and discussions can be accessed in the paper linked in the beginning):
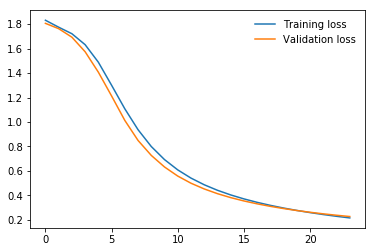
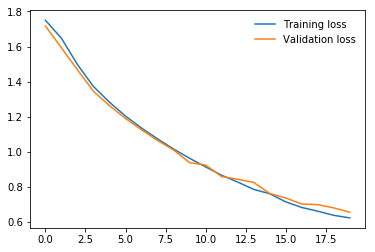
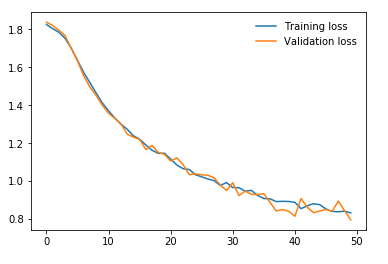
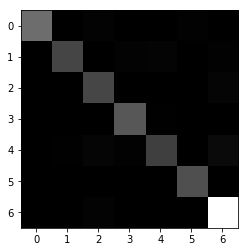
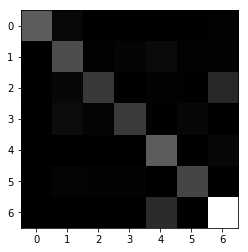